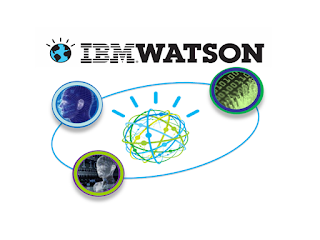
There are many
data analytics tools. In my previous post, I wrote briefly about Tableau Software.
In this post, I will summarize other type of data analytics tools which is IBM
Watson. IBM Watson built to deal with huge amount of data. It also built to be
as question answering machine and it has the ability to understand the natural
language which lead to create more natural relationship between humans and computers.
Watson create a response by depending on generating hypothesis and evaluation. Watson
understands the knowledge of the data and applies it to the problem,
alternative solutions provided and underlying evidence to support each alternative.
“Watson has a unique feature which is the ability to learn a repeated use”. By
tracking users feedback and from its success and failures, Watson become more
smarting and presenting new information. Watson is not a replacement of human
experts it is a supportive system to help creating decisions out of huge volume
of unstructured data.
IBM Watson has a
lot of advantages such as:
-
Ability
to deal with massive quantities of data
-
For making
decisions, it acts as a supportive system and improve performance
-
Ability
to process unstructured data
Some limitations
that IBM Watson has that:
-
It is
only available in English language
-
It processes
structured data indirectly
-
With
limited sources, Watson increases the rate of data
-
It is
costly system which targeting only big organizations
IBM Watson implements
in different fields such as:
-
Healthcare:
Watson is used at M.D. Anderson Cancer Center in Houston to help them diagnose
and develop treatments for patients through searching in database which include
doctors’ notes and other medical papers.
-
Non-profits:
by using natural language founders and donors has the opportunity to ask Watson
questions and receive instant answers.
To read more about IBM Watson click here